What We Know—and Don’t Know—About AI and Regulation
August 24, 2023
Amid current clarion calls for the regulation of artificial intelligence (AI)—or, more precisely, machine learning (ML)—is the assumption (or in some cases blind faith) that regulation can (and will) keep people safe from possible harms due to the technology’s use. The fact that the very creators of ML applications make some of these calls adds credence to the belief that we can take actionable steps, and that what that something is can be easily defined and implemented. That certainly appears to be the case with the European Union’s AI Act and Canada’s Artificial Intelligence and Data Act.
A key feature of both the EU and Canadian legislation is the obligation to define and identify the risks AI applications pose and ensure that appropriate risk mitigation strategies are put in place and continually monitored. As the promotors of the Canadian legislation claim, “For businesses, this means clear rules to help them innovate and realize the full potential of AI. For Canadians, it means AI systems used in Canada will be safe and developed with their best interest in mind.”
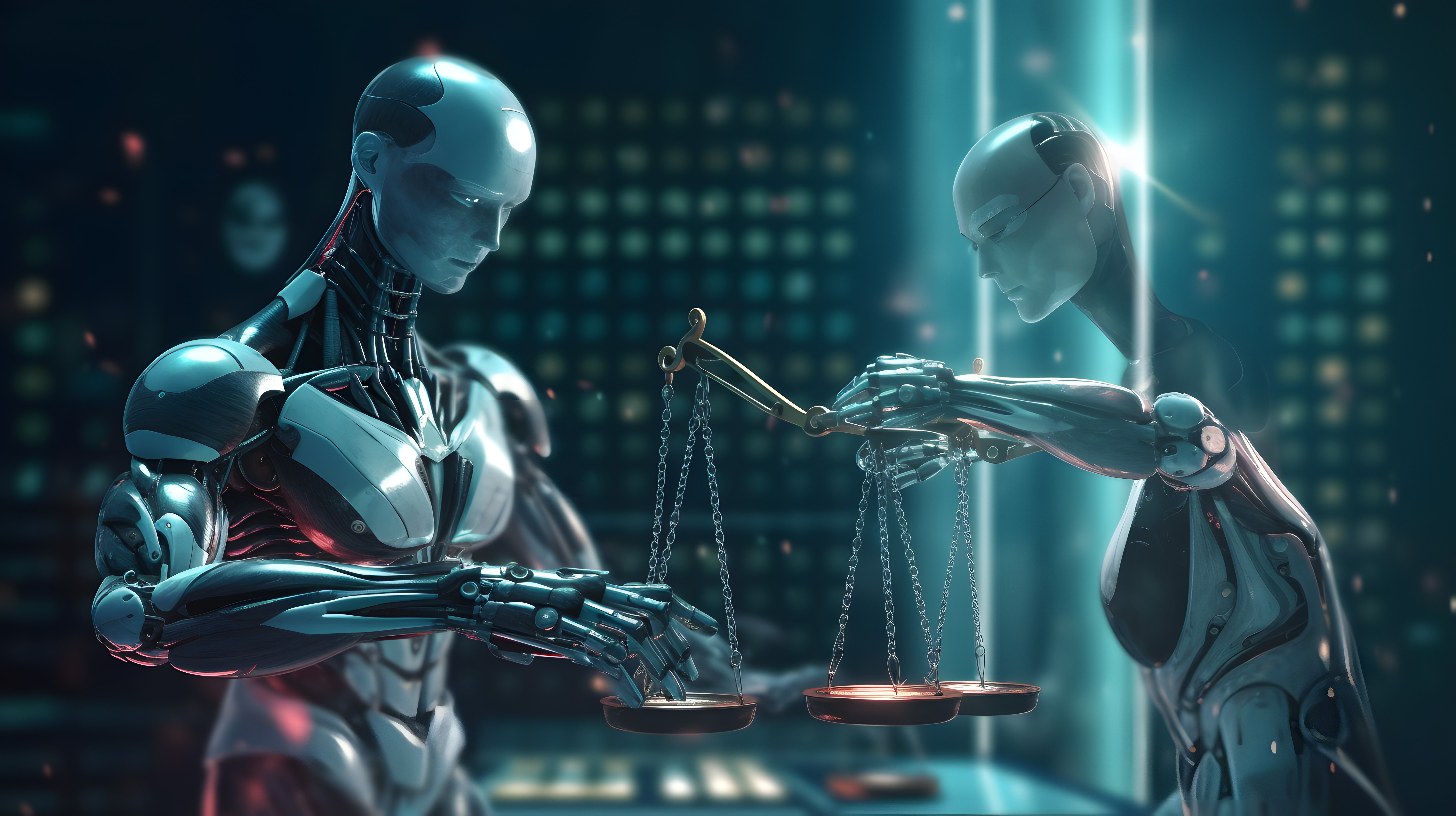
However, as former Federal Trade Commissioner Maureen K. Ohlhausen observed, drawing on Friedrich Hayek’s “The Use of Knowledge in Society,” regulation is a task that needs to be approached with a healthy dose of regulatory humility—that is, recognition of regulation’s inherent limitations. A regulator must acquire knowledge about the present state and future trends of the industry’s regulation. The more prescriptive the regulation and the more complex the industry, the more detailed knowledge the regulator must collect.
But this supposes that the relevant information is already known, or can be known, in the first place. And importantly, that the regulator acknowledges what is not already known, and maybe cannot ever be known, will influence the ability to craft and enforce effective regulations. Failing to acknowledge this factor leads either to falling for Daniel Kahneman’s “what you see is all there is” cognitive bias or, in Ohlhausen’s view, overconfidence in the regulator’s ability to use regulatory means to achieve desired objectives. The less the regulator knows, or the more that cannot be known by the regulator or anyone else, the greater the likelihood that the regulation itself will impose harm, over and above any harm that may be caused by the subject of that regulation.
Therefore, a distinction between risk and uncertainty is crucial for understanding regulatory humility. Frank Knight articulated in his 1921 book Risk, Uncertainty and Profit (Beard Books) that “a known risk is easily converted into an effective certainty” using probabilities, while “true uncertainty is not susceptible to measurement.” John Kay and Mervyn King provide a modern interpretation in Radical Uncertainty: Decision-Making Beyond the Numbers (W.W. Norton & Company, 2020.) They distinguish between the state of radical uncertainty or complexity (order is not apparent ex ante so no certainty of outcomes can be anticipated [Knightian uncertainty]) and the merely complicated (with sufficient time, information, and resources, order can be discerned and probabilities attached, leading to a state of Knightian risk). They term the quest for understanding the latter cases “puzzles”—for which there is one or more potential solutions—and the former, “problems”—where there is no clearly defined or obtainable solution, no matter how much effort is exerted.
What then, does this mean for the so-called risk-based approaches to regulating ML proposed for the EU and Canada?
At the nub of the ML problem is that the current state of knowledge about ML applications and their likely effects in various sectors is scant, even amongst those developing them. A clear and precise definition of what constitutes ML appears almost impossible to pin down. This is a state of Knightian uncertainty: Definitions and outcome probabilities are nearly impossible to assign, not to mention the probabilities of specific interventions having quantified likelihoods of success. By Ohlhausen, Hayek, Knight, Kay, and King, the AI/ML situation is not one amenable to regulation relying on principles of risk and risk management. A dose of regulatory humility is required.
A closer examination of the EU regulations reveals it is not that the risks of ML, per se, are being managed, but rather the sectors where it is feared that any potential harms will be more costly or irreversible are being ring-fenced and sheltered from uncertainty. And to the extent that some firms by dint of their size might possibly cause more instances of harm with their applications, they too face additional restrictions as regulators try to shift costs of uncertainty onto those with pockets big enough to bear the insurance premium of uncertainty for society.
Applying a good dose of humility thus leads to the conclusion that this movement is not regulating the risks of ML but rather managing the consequences of the fear of the uncertain.
Sign up for AEI’s Tech Policy Daily newsletter
The latest on technology policy from AEI in your inbox every morning